Dimensionality reduction
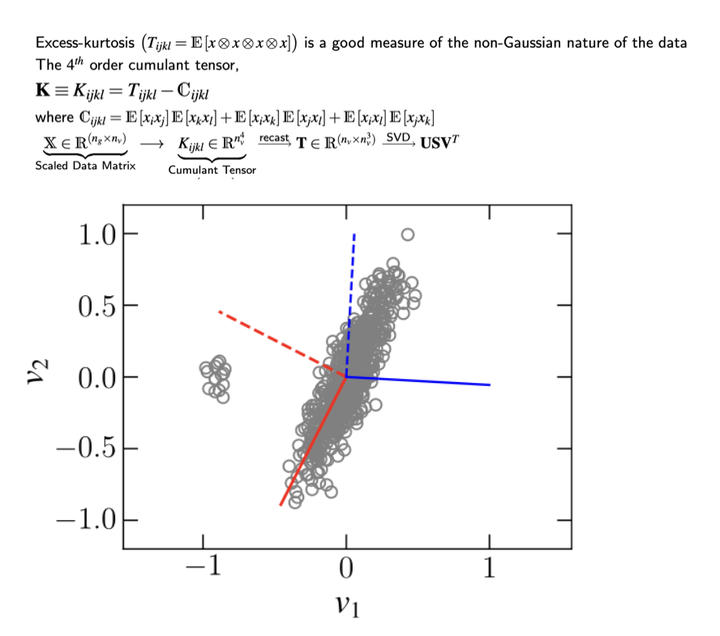
Direct numerical simulations (DNS) of turbulent reacting flows, which capture detailed chemical kinetics and their interactions with turbulent structures, demand massive computations and are often performed on large supercomputers. Current state-of-the-art simulations use chemical mechanisms that comprise of tens of species participating in tens or hundreds of reactions. A valuable approach in reducing the associated computational expense involves identifying lower-dimensional manifolds, i.e., a smaller subset of representative thermo-chemical scalars to express, and solve the conservation equations. Principal Component Analysis (PCA) is a popularly employed to identify the low-dimensional maniold and used as a dimensionality reduction technique in numerical simulations of combustion processes. However, it could be argued that the co-variance eigenvectors of the thermo-chemical state space used for PCA may be unable to fully capture information about important, extreme-valued localized chemical dynamics like ignition-kernel-formation. In such situations, a useful approach may be to consider a dimensionality reduction procedure where the required principal vectors are computed from high-order joint statistical moments and may enable improved identification of the state space directions corresponding to stiff dynamics. In this project, we explore one such procedure, namely the co-kurtosis PCA (CoK-PCA), that is based on the fourth-order joint statistical moment i.e. the co-kurtosis tensor. Apriori analysis of combustion datasets corresponding to spontaneous ignition of premixed ethylene-air in a simple homogeneous reactor and ethanol-fueled homogeneous charged compression ignition (HCCI) engine demonstrates that the co-kurtosis based reduced manifold is able to represents the original thermo-chemical state in the regions where chemical reactions are important with significant accuracy.
References:
- Jonnalagadda, A., Kulkarni, S., Rodhiya, A., Kolla, H. and Aditya, K., 2023. A co-kurtosis based dimensionality reduction method for combustion datasets. Combustion and Flame.