Medical Imaging Group Brochure
Image Reconstruction:
Quantitative Photoacoustic Imaging:
The photoacoustic imaging has a distinct advantage of being scalable to image both micro and macro-level tissue structures, where it combine light and sound. The emphasis on photoacoustic imaging is to obtain initial pressure map, which gives the signatures of absorption coefficient of the tissue under investigation. Till now, the image reconstruction methods that are available are more qualitative in nature, the emphasis here will be to develop quantitative image reconstruction methods especially in cases where only limited-data is available.MRI-DOT:
There is a need to develop robust and computationally inexpensive 3D diffuse optical tomographic image reconstruction methods that use spatial priors from conventional imaging modalities (MRI, CT, and Ultrasound). These 3D methods must improve the quantitative and qualitative accuracy of optical images due to the additional information available from conventional imaging modalities. Even though these methods are currently readily available for 2D, these have not been translated into 3D due to mainly computational complexity and insufficient boundary data. A generalized framework that can be used in 3D will be developed such that one can easily incorporate spatial priors. Moreover, optimal 3D data collection geometries will also be explored to see the effect of boundary measurements on the image quality and quantification of optical properties through a series of numerical experimentsMRI-NIR Mesh Analysis:
![]() |
![]() |
Original
MRI-NIR
Mesh |
Simplified
MRI-NIR
mesh |
In Collaboration with Vijay Natarajan, Ph.D.
Dynamic Diffuse Optical Tomography (DOT):
Near-infrared (NIR) diffuse optical tomography uses a finite set of boundary measurements made on the soft-tissue using light in the spectral range of 600-1000 nm to reconstruct the internal distribution of optical properties. The NIR optical tomography has been most intensively investigated for breast cancer imaging and brain function assay. As the malignant tumor vasculature is known to be different from the benign one, the main aim of the NIR optical tomography has been to non-invasively capture the difference in the vasculature or, more precisely, the dynamics of it as NIR light can penetrate deep tissues. Efforts have been made to develop NIR systems capable of acquiring the NIR tomography data at high speed up to the video-rate. Although the data acquisition in these rapid dynamic (video-rate) NIR optical tomography systems has been performed at higher rates (~ 35 frames/sec), the image reconstruction has always been handled off-line due the computational complexity of iterative algorithms. Typical speed of 10 seconds per frame to recover the distribution of absorption coefficient is reported in the literature by using a coarse mesh on a Pentium IV 2 GHz CPU. Even though there are methodologies for real-time image reconstructions (integrated with the data acquisition), they often require high end workstations that have the capability of parallel processing of these algorithms.This work developed and presented a Singular Value Decomposition (SVD)-based computationally efficient iterative algorithm that can, in principle, obtain the video-rate optical tomographic image reconstruction in-line with the data acquisition. These studies indicate that the performance of SVD-based algorithm in terms of contrast recovery (quantitation of optical images) is better compared to nonlinear iterative (conventional) algorithms, provided the initial guess is close to the actual solution. The nonlinear algorithms can provide better quality images compared to the SVD-based algorithms. Moreover, the analytical and numerical equivalence of the SVD-based algorithm to linear iterative algorithms was also established as a part of this work. It is also demonstrated that the SVD-based image reconstruction typically requires O(NN2) operations per iteration, as contrasted with linear and nonlinear iterative methods that, respectively, require O(NN3) and O(NN6) operations, with “NN” being the number of unknown parameters in the optical image reconstruction procedure.
Saurabh Gupta, Phaneendra K. Yalavarthy, Debasish Roy, Daqing Piao, and Ram M. Vasu, “Singular Value Decomposition based computationally efficient algorithm for rapid dynamic near-infrared diffuse optical tomography,” Med. Phys. 36(12), 5559-5567 (2009). PDF
Signal/Image Analysis:
Lung Segmentation:
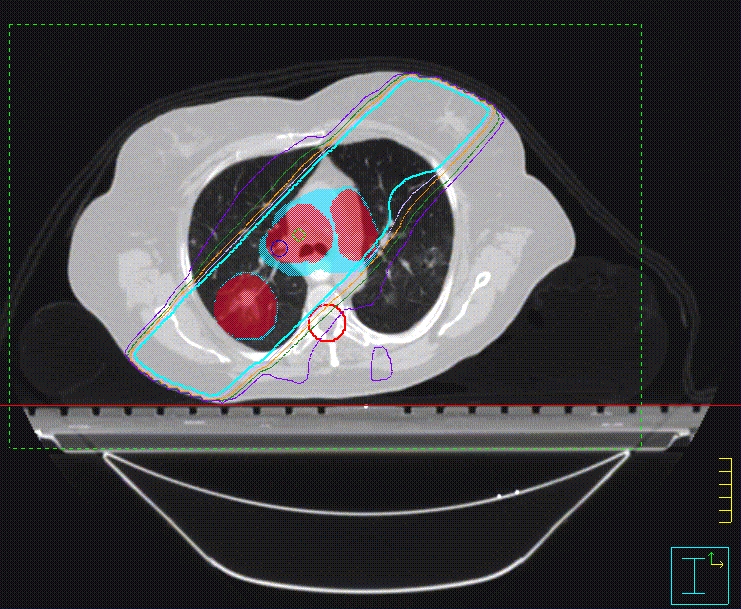
Automated lung segmentation in computed tomography scans is a precursor to most pulmonary computer-aided diagnostic (CAD) methods. This work explored mainly two automated methods for performing the lung segmentations, first being dynamic threshold method and second being level-set method. First method has three main steps. First, the lung region is extracted from the CT images by gray-level thresholding. Then, identifying the anterior and posterior junctions by dynamic programming separates the left and right lungs. In second method based on level-set method, we evolve a contour on CT image starting level-set of order 0 for typical 200 iterations. This gives well-defined contours for lungs along with other parts in the image. In both these methods, a sequence of morphological operations is used to smooth the irregular boundary along the mediastinum in order to obtain results consistent with those obtained by manual analysis, in which only the most central pulmonary arteries are excluded from the lung region. Both these methods has been tested by processing CT datasets from 40 normal subjects and found that root mean square difference between the automated and human analysis is 2 pixels (1 mm). The dynamic threshold method was computationally faster compared to level-set method.
Detection of masses in Mammograms using advanced image processing techniques.
Breathing Signal Analysis:
The usage of multi-row
detectors in computed tomography (4D CT) has lead to
obtaining anatomical images of lungs with a temporal
resolution of 4.2 msec. Typically the 4D CT data is
obtained continuously over 3-4 breathing cyles (for a
duration of ~ 20 sec) in multiple intervals and they are
retrospectively gated to obtain 4D CT images to study the
lung physiology. This 4D CT is currently used only on
oncological patients because of retrospective gating
(which result in higher dose). By accurately predicting
the breathing signal, it is possible to prospectively gate
CT and obtain these 4D CT images. Efforts are underway to
use Autoregression and Moving Average (ARMA) Models and
Ensemble Kalman Filters to extrapolate the breathing
signal that could be used for prospective gating. If these
models are proven to be successful, this will lead to
usage of 4D CT in most cases of pulmonary diseases (such
as asthma).