Chest computed tomography (CT) imaging has become indispensable for staging and managing COVID-19, and current evaluation of anomalies/abnormalities associated with COVID-19 have been performed by the visual score at present. This evaluation requires important step of classifying the severity of COVID19 infection as mild, moderate, and severe. The development of automated methods for quantifying COVID-19 abnormalities in these CT images is invaluable to clinicians to choose a treatment plan and also to know the patient’s response to the treatment. This is the first method that provides automated segmentation providing a quantitative measure of COVID19 related lung infection using a light-weight CNN. CDS researchers in collaboration with Oslo University Hospital and University of Agder, Norway, have proposed anamorphic depth embedding based light-weight CNN, called Anam-Net, to segment anomalies in COVID-19 Chest CT images. The developed model is deployable in mobile platforms and an Android application called CovSeg was developed to remove the requirement of having specialised hardware to run this AI model.
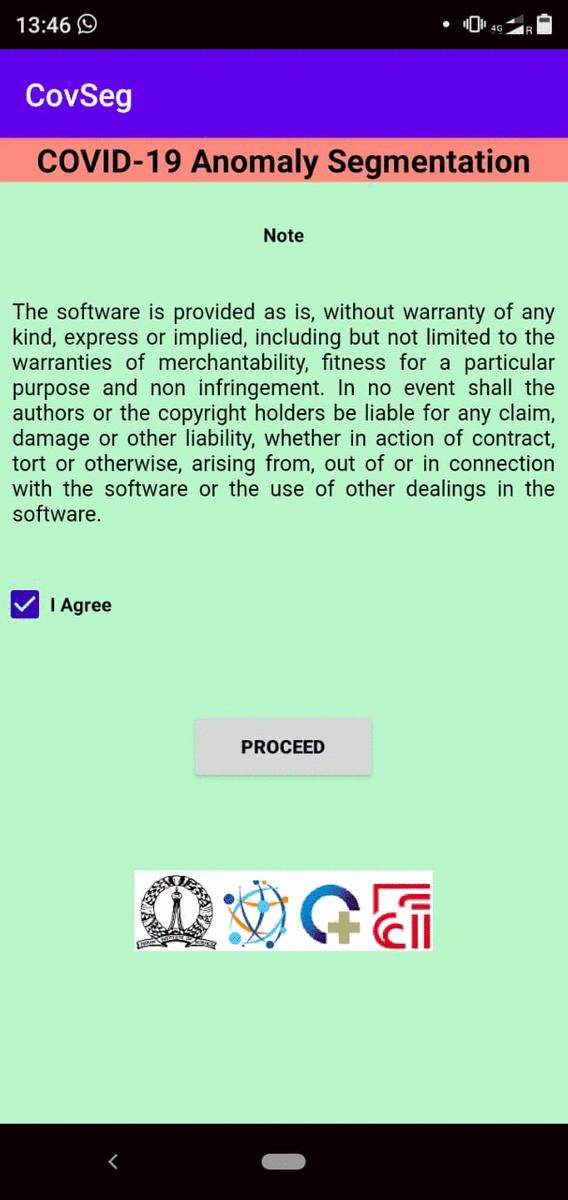
Citation:
Naveen Paluru, Aveen Dayal, Havard B. Jenssen, Tomas Sakinis, Linga R. Cenkeramaddi, Jaya Prakash, and Phaneendra K. Yalavarthy, “Anam-Net: Anamorphic Depth Embedding based Light-Weight CNN for Segmentation of Anomalies in COVID-19 Chest CT Images,” IEEE Transactions on Neural Networks and Learning Systems (Fast Track: COVID-19 Focused Papers) 2021 (in press). [preprint is available @ https://cds.iisc.ac.in/faculty/yalavarthy/Publications.html]
Project Repository: https://github.com/NaveenPaluru/Segmentation-COVID-19